๋ฅ๋ฌ๋ ๊ฐ์ lec 03
ML lab 03 - Linear Regression์ cost ์ต์ํ์ TensorFlow ๊ตฌํ (new)
import tensorflow as tf
import matplotlib.pyplot as plt
Variables Initialize
X = [1, 2, 3]
Y = [1, 2, 3]
W = tf.placeholder(tf.float32)
Hypothesis
#hypothesis = Wx
hypothesis = X * W
# cost = avg(sum(H - y)2)
cost = tf.reduce_mean(tf.square(hypothesis - Y))
Session start
sess = tf.Session()
sess.run(tf.global_variables_initializer())
W_val = []
cost_val = []
for i in range(-30, 50):
feed_W = i * 0.1
curr_cost, curr_W = sess.run([cost, W], feed_dict={W: feed_W})
W_val.append(curr_W)
cost_val.append(curr_cost)
Visualize
plt.plot(W_val, cost_val)
plt.show()
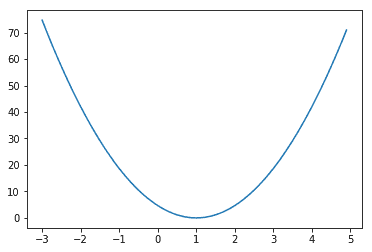
Gradient descent
์ฐ๋ฆฌ์ cost ๊ฐ์ด ๋ฐฅ๊ทธ๋ฆ ๋ชจ์์ ๊ทธ๋ํ์ผ ๋ ์ฌ์ฉํ๋ ์๊ณ ๋ฆฌ์ฆ ๊ฐ์ฅ ์์ชฝ์ (1,0)์ ์ ์ ์ฐพ๋ ๊ฒ์ด ๋ชฉํ
๋ฏธ๋ถ : ํ ์ ์ ๊ธฐ์ธ๊ธฐ๋ฅผ ๋ํ๋
โ ๋ชจ์์ ๊ฒฝ์ฐ +
โ ๋ชจ์์ ๊ฒฝ์ฐ -
์์ ๊ฐ์ ๊ทธ๋ํ์์ (4, 50)์ ๊ฒฝ์ฐ
โ ๋ชจ์์ด๋ฏ๋ก + W๊ฐ 1์ด ๋๊ธฐ ์ํด์ -๋ก ์์ง์ฌ์ผํจ
์์ ๊ฐ์ ๊ทธ๋ํ์์ (-2, 40)์ ๊ฒฝ์ฐ
โ ๋ชจ์์ด๋ฏ๋ก - W๊ฐ 1์ด ๋๊ธฐ ์ํด์ +๋ก ์์ง์ฌ์ผํจ
์์์ผ๋ก ๊ตฌํ ์
๋ฏธ๋ถํ ๊ฒฐ๊ณผ
learning_rate = 0.1
gradient = tf.reduce_mean((W * X - Y) *X)
descent = W - learning_rate * gradient
update = W.assign(descent)
Gradient descent ์ ์ฉ
import tensorflow as tf
x_data = [1, 2, 3]
y_data = [1, 2, 3]
W = tf.Variable(tf.random_normal([1]), name = 'weight')
X = tf.placeholder(tf.float32)
Y = tf.placeholder(tf.float32)
hypothesis = X * W
cost = tf.reduce_sum(tf.square(hypothesis - Y))
learning_rate = 0.1
gradient = tf.reduce_mean((W * X - Y) * X)
descent = W - learning_rate * gradient
update = W.assign(descent)
sess = tf.Session()
sess.run(tf.global_variables_initializer())
for step in range(21):
sess.run(update, feed_dict={X: x_data, Y: y_data})
print(step, sess.run(cost, feed_dict={X: x_data, Y:y_data}), sess.run(W))
0 4.66668 [ 0.42264879]
1 1.32741 [ 0.69207931]
2 0.377575 [ 0.83577561]
3 0.107399 [ 0.91241366]
4 0.0305491 [ 0.9532873]
5 0.00868952 [ 0.97508657]
6 0.00247167 [ 0.98671287]
7 0.000703047 [ 0.99291354]
8 0.000199983 [ 0.99622053]
9 5.68846e-05 [ 0.99798429]
10 1.61796e-05 [ 0.99892497]
11 4.60181e-06 [ 0.99942666]
12 1.30917e-06 [ 0.99969423]
13 3.72323e-07 [ 0.99983692]
14 1.05845e-07 [ 0.99991304]
15 3.01388e-08 [ 0.99995363]
16 8.55728e-09 [ 0.99997526]
17 2.43397e-09 [ 0.99998683]
18 6.87535e-10 [ 0.99999297]
19 1.96071e-10 [ 0.99999624]
20 5.89608e-11 [ 0.99999797]
Minimize : Gradient descent
optimizer = tf.train.GradientDescentOptimizer(learning_rate = 0.1)
train = optimizer.minimize(cost)
import tensorflow as tf
X = [1, 2, 3]
Y = [1, 2, 3]
#W = tf.Variable(5.0)
W = tf.Variable(-45.0)
hypothesis = X * W
cost = tf.reduce_mean(tf.square(hypothesis - Y))
optimizer = tf.train.GradientDescentOptimizer(learning_rate=0.1)
train = optimizer.minimize(cost)
sess = tf.Session()
sess.run(tf.global_variables_initializer())
for step in range(10):
print(step, sess.run(W))
sess.run(train)
0 5.0
1 5.0
2 5.0
3 5.0
4 5.0
5 5.0
6 5.0
7 5.0
8 5.0
9 5.0
Optional: compute_gradient and apply_gradient
GradientDescentOptimizer๋ฅผ ์๋ก์ด ์์ผ๋ก ์ปค์คํ ๋ง์ด์ง ํ๊ณ ์ถ์ ๋ ๋ฐฉ๋ฒ
import tensorflow as tf
X = [1, 2, 3]
Y = [1, 2, 3]
W = tf.Variable(5.0)
hypothesis = X * W
gradient = tf.reduce_mean((W * X - Y) * X) * 2
cost = tf.reduce_mean(tf.square(hypothesis - Y))
optimizer = tf.train.GradientDescentOptimizer(learning_rate=0.1)
gvs = optimizer.compute_gradients(cost)
apply_gradients = optimizer.apply_gradients(gvs)
sess = tf.Session()
sess.run(tf.global_variables_initializer())
for step in range(10):
print(step, sess.run([gradient, W]))
sess.run(apply_gradients)
0 [37.333332, 5.0]
1 [2.4888866, 1.2666664]
2 [0.16592591, 1.0177778]
3 [0.011061668, 1.0011852]
4 [0.00073742867, 1.000079]
5 [4.895528e-05, 1.0000052]
6 [3.0994415e-06, 1.0000004]
7 [0.0, 1.0]
8 [0.0, 1.0]
9 [0.0, 1.0]